High quality products made easier
Control + Reproduce + Validate + Trace + Expose
Our mission is to make it easier to design & build high quality products.
Our Product Life Cycle & Manufacturing (PLCM) software lets you build better products by giving a controllable and reproducible manufacturing process:
- Operators receive clear on-screen instructions.
- All steps and measurements are logged and visualized.
- Dashboards give full insight into actual and historical status.
The PLCM makes quality easier because the process descriptions and work instructions are all edited in shared documents in a web based environment, ensuring a single source of truth.
The process design tool allows for easy configuration of product manufacturing steps. Adding Materials, Machinery & Tools and Test Methods is a breeze. This ensures the validation of inputs and outputs is accurate. Additionally, the process designs created can be reused multiple times, resulting in a more efficient and cost-effective New Product Introduction (NPI).
The advanced dashboard technology offers real-time visibility into Work-in-Progress. This allows for accurate and instant overviews of production data, as well as clear display of quality figures. The system also features easy access to specific details on production orders with just one click. The way the data is exposed aids in decision making and identifying areas of improvement in the production process.
The user interface is intuitive. In one glance a user sees where he is in the whole process. The process sequence is enforced, assuring a full control and consistent execution. Work instructions are integrated in the same user interface ensuring the correct information is presented at the right process step.
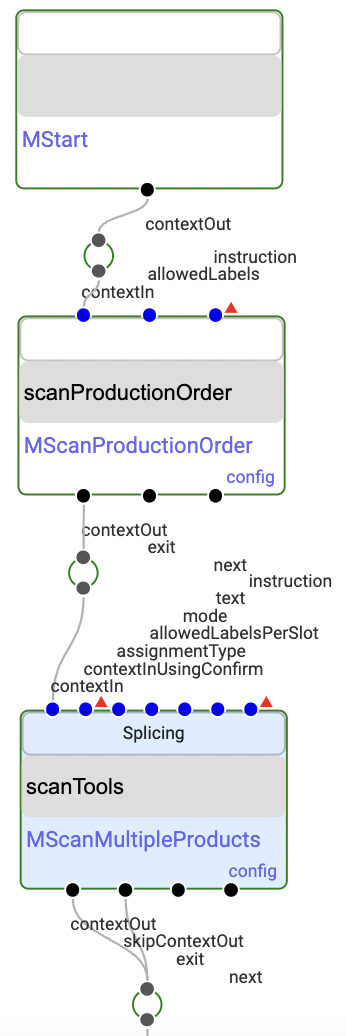
The software gives a controlled and reproducible manufacturing process, based on descriptions using simple diagrams. An explicit, detailed recipe is used together with clear instructions for operators.
This ensures that the exact steps as required are followed when manufacturing; nothing gets skipped, no wrong steps are taken.
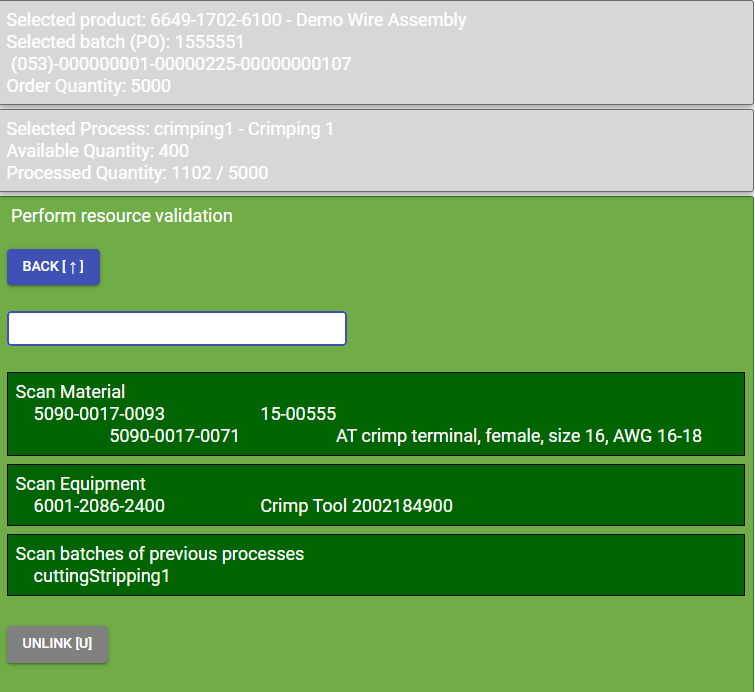
Materials and tools are specified up-front and scanned and traced during operation. Up front configuration determines which operator roles are allowed to perform which operations.
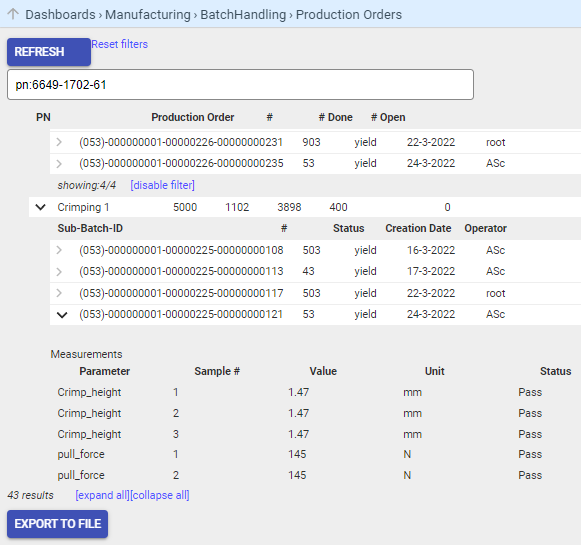
Dashboards show the WIP status, at various levels of granularity; from a high level production overview down to the measurement details of a single produced item.
All data is protected against ransomware attacks, errors and disasters by:
- Archiving data every second in such a manner that it cannot be deleted.
- Marking deletions but keeping underlying data whenever a user deletes something.
- Replicating data across zones in the primary data center, and across data centers that are more than 100 km apart.